Book review: Statistics for Health Data Science: An Organic Approach
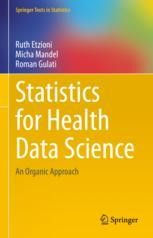
Ruth Etzioni, Micha Mandel, Roman Gulati
Springer, January 2021
Statistics for Health Data Science is a well-written book that covers a wide range of topics. The authors state that the book is appropriate for someone with an introductory course in statistical models and I found that audience to be appropriate as I read the text. The basics of statistical inference and linear regression modeling are reviewed briefly in Chapters 2 and 3 before more advanced material is introduced. The middle third of the book covers models for binary and count data, and health care costs (including gamma models and two-part models for continuous outcomes with a high number of zeros). Finally, the book ends with multiple chapters on more advanced topics: bootstrap, causal inference, complex surveys, and prediction. In general, the book is very easy to read and provides many easy-to-understand and well-developed examples.
The “Organic Approach” the authors take motivates all statistical models by real-world examples, often using publicly available data. Equations are used sparingly but when they are, they are fully developed and not notation-heavy. The authors stress that the research question should guide the decisions made in the statistical analysis. While chapters on causal inference and prediction may make that obvious, these topics are broached well before their respective chapters. For example, in the overview of linear regression in chapter 3, variable selection is discussed from standpoints of both exploratory and theoretically-driven questions. The authors explain how these approaches may lead to different analytical choices and the pros and cons of each approach in each context.
The authors’ website includes all code needed to reproduce the examples discussed in the book, allowing the reader to follow along and providing coding examples to use in future analyses. I see the decision to keep coding out of the text is a good one in this case – the book is heavily focused on understanding statistical concepts behind the techniques rather than applied coding. Long examples with excessive coding may have hindered the authors in achieving their goal. The only downside of this book from an instructor’s standpoint might be the lack of any end-of-chapter exercises.
I thoroughly enjoyed reading this book and am a bit disappointed that I don’t teach a class in which it would fit well. The closest course I teach covers linear and logistic regression, which are only two of the ten chapters in the book. I would, however, highly suggest it to any of my students who will be taking additional classes in the more advanced topics the book covers. The authors do a great job distilling important statistical information into a compact presentation and provide a very natural (“organic”?) development from one topic to the next. Such a book would be a good companion to the more technically detailed books focused on the topics this book covers in a single chapter. While I may not be assigning this book for my class, the quality of the text makes me reconsider the content I cover and whether I could make it work. At the least, I will surely be referring to this text as I prepare to teach in the fall.
Comments