Fall Webinar recap: Teaching Causal Inference
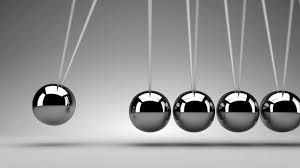
[Editor's note: This webinar recap was written many months ago, following the Fall 2020 webinar. Due to an administrative oversight, it's publication to the blog was delayed until now.]
Why? Because! Or Maybe Not.
“Give a man a fish and you feed him for a day. Teach a man to fish and you feed him for a lifetime.” Most of us have heard this before. Teaching someone how to do something can be more beneficial in the long run than doing it for them. This is the goal we educators aim to achieve. The difficult part, however, can be finding the right balance so that students can “see the forest for the trees.” Students should not be so focused on the details of a problem that they miss the big picture.
Dr. Douglas Landsittel of the University of Pittsburgh described such challenges and offered educational strategies related to teaching causal inference in the health sciences in this webinar: “Challenges in and Educational Strategies for Teaching Causal Inference in the Health Sciences”.
A professor in biomedical informatics, Dr. Landsittel has taught in person and online courses in comparative effectiveness research (CER) and patient-centered outcomes research (PCOR). His biomedical statistics and data science lab focuses on developing CER/PCOR educational resources. Dr. Landsittel has taught statisticians, epidemiologists, clinical researchers, biomedical researchers, and other graduate or post-graduate researchers the foundational issues in concepts, designs and mathematics, discipline-specific advanced methods, and causal inference.
The Forest (The Big Picture)
As Dr. Landsittel acknowledged, critics have claimed that teaching causal inference to clinical researchers may be too complicated and provide just enough knowledge to be dangerous, recommending that they hand it over to statisticians. In response, Dr. Landsittel’s webinar focuses on teaching causal inference to clinical researchers. In addition to CER, Dr. Landsittel addressed efficacy of interventions and medical treatments, health disparities research, and evaluating sex as a biological variable.
Dr. Landsittel has taught mostly MS and MD students, post docs, and faculty whose areas of research and statistical skill sets differ. Their objective is to develop a concept proposal in CER including the research question, study design, engagement of the stakeholder, heterogeneity of effects, and the basics of causal inference.
Dr. Landsittel’s primary goal is to help his students build an appreciation of causal inference, to recognize the significance and complexity of causal inference, and to describe concepts of potential outcomes, target trials, causal roadmaps, pseudo randomization using minimal statistical methods with emphasis on assumptions and challenges and underlying mathematics. He provides an insight on where to look for more information with website resources and introduces flexibility through a project proposal format.
Early in the course, Dr. Landsittel introduces the frameworks for causal inference and emphasizes key details repeatedly, including potential outcomes, the Target trial (based on an observational data set, from which they develop a research question, inclusion and exclusion criteria, causal contrast and estimand, and emulate a hypothetical trial), and walks through the Causal roadmap of Peterson and van der Laan (who propose a 7-step process).
Dr. Landsittel recommended thinking of causal inference methods as an extension of standard methods and covering other topics such as propensity score-based methods, instrumental variables, and the difference between point and sustained interventions.
The Trees (Some Details)
In sharing his personal view for this audience, Dr. Landsittel noted the importance of the following:
- conducting all analyses is counter-productive, rather they should gain the knowledge for where these methods are applicable so they can communicate with statisticians with whom they work
- having shared knowledge is mutually beneficial
- being aware of the variability in the skillsets of collaborators is important
- accept that one’s role differs greatly across projects
- avoid being judgmental but openly present concerns and limitations.
As an educator, Dr. Landsittel emphasizes design and encourages causal graphs. He describes assumptions and details addressing variations in causal estimands, positivity, SUTVA, and conditional independence.
The Landsittel Lab
For the remainder of the webinar, Dr. Landsittel took us on a virtual tour of his Biomedical Statistics and Data Science Lab. We encourage you to explore the Educational Resources, where you will find three online tools for comparative effectiveness and causal inference:
- Online Self-Guided Course: PCOR Studies
- Online Self-Guided Course: Propensity Score-Based Methods
- DECODE CER Tool
We welcome your thoughts in the comments below.
Comments