Review of Modelling Survival Data in Medical Research
Resource Review Post by Ming Hu from Mayo Clinic
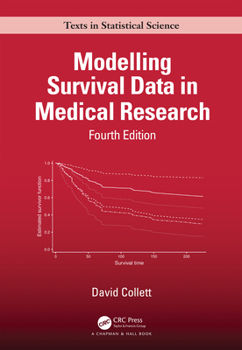
David Collett's "Modelling Survival Data in Medical Research," now in its fourth edition released not long ago in 2023, offers a comprehensive exploration of survival analysis in the context of medical research. The book is structured into 17 chapters, with an emphasis on practical applications at an intermediate level. It covers fundamental concepts in survival analysis, introduces the Cox regression model, explores parametric models, and addresses various extensions to basic models. This newest fourth edition incorporates chapters covering topics such as interval-censored survival data, joint modeling of longitudinal and survival data, and piecewise exponential models.
The core of the book comprises Chapters 1 to 7, beginning with an introduction to the basic concepts in survival analysis in Chapter 1. Chapter 2 delves into methods for summarizing and comparing survival data across different groups. Chapter 3 introduces the modeling approach, providing an in-depth presentation of the Cox regression model. Following this, Chapter 4 addresses methods for checking the adequacy of a fitted model. Chapter 5 explores parametric proportional hazards models and accelerated failure time models, followed by Chapter 6, which focuses on flexible parametric models. Chapter 7 then presents model-checking diagnostics for parametric models. Subsequent chapters extend the foundational models, covering topics such as time-dependent variables (Chapter 8), analysis of interval-censored data (Chapter 9), and frailty models incorporating random effects (Chapter 10). Chapter 11 summarizes techniques for non-proportional hazards assumptions, facilitating, for example, comparisons of outcomes across institutions. Chapter 12 introduces competing risk models, accommodating various causes of death, while Chapter 13 extends the Cox regression model to handle multiple events via event history analysis. Chapter 14 provides methods for analyzing data with dependent censoring, and Chapter 15 guides readers on determining sample size requirements for studies involving survival time outcomes. The final two chapters, Chapters 16 and 17, detail Bayesian methods and the practical use of the R software, respectively. Together, these chapters offer a comprehensive journey through survival analysis, from foundational concepts to advanced modeling techniques and practical implementation.
This book is tailored for a diverse audience, including students in MPH/MS programs, clinicians, and professionals in the health sciences. While the author assumes a basic knowledge of statistical methods and familiarity with linear regression analysis, there is no requirement for expertise in more modern data-intensive methods. Collett ensures accessibility by minimizing references in the text and providing bibliographic notes and further reading suggestions at the end of each chapter. The book is designed to meet the needs of those in the medical field, balancing methodological detail with practical examples and exercises, making it a valuable resource for both beginners and experienced practitioners in medical research and statistical analysis.
Critically assessing the book, one noticeable gap is the limited discussion on the integration of more recent artificial intelligence (AI) tools in the practical implementation of survival analysis. Given the increasing role of AI in medical research and data science, a section on its applications would enhance the book's relevance to contemporary practices. Furthermore, while the additional Chapter 17 on actual R implementations is a huge step towards making the book more accessible for readers who are looking for a guidebook on carrying out data analysis on real-world projects, it is debatable whether this separate chapter would be equally convenient in a classroom setting.
In terms of educational use, "Modelling Survival Data in Medical Research" is highly valuable for journal clubs and graduate programs in data science, especially those with a focus on health sciences. The abundance of illustrations and carefully worked, real-data examples make the book an excellent supportive material resource. The book's intermediate level and practical orientation ensure it is suitable for both introductory and somewhat more advanced courses in survival analysis.
In conclusion, Collett's book impressively covers essential theory, providing practical insights and tips for those involved in medical and pharmaceutical research. It serves not only as a quick reference for statistical practitioners but also as a potential textbook for graduate-level survival analysis courses. The incorporation of R software and the emphasis on applied components make it convenient for lecturers seeking to integrate practical elements into post-graduate statistics or operational research degree courses. Continuing on earlier editions, which have been highly praised by reviews in the Journal of American Statistical Association, this book stands as a valuable resource for anyone seeking a comprehensive understanding of survival analysis in a medical context.
Comments